AI in loyalty marketing and loyalty programs
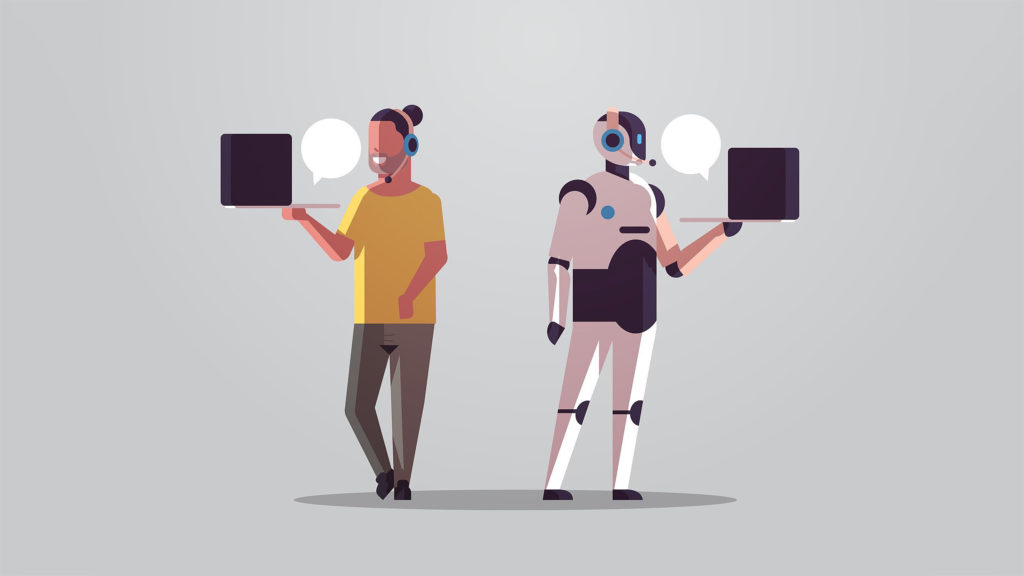
AI is much talked about. It has the potential to address a lot of business challenges, and enable many forms of elusive innovation in loyalty marketing.
The biggest opportunities for loyalty programs relate to operating more efficiently to reduce cost, and improving personalization.
Most larger loyalty programs have been experimenting with artificial intelligence for years now, and value has been found around personalization and fraud management. For smaller and medium-sized enterprises, artificial intelligence-based solutions have been difficult to embrace – until recently.
New AI platforms are overcoming cost and complexity barriers, and are now being applied to a much wider range of purposes.
This article will cover the key opportunities for AI in loyalty – and more widely in the travel and retail sectors. These opportunities are largely in four key areas:
- Inventory, pricing & promotions – making use of available data in order to sell the right product to the right person at the right price, with improved profitability. In loyalty, this can be especially powerful in using distressed inventory to create greater customer value for members, in adjusting margins based on demand, or proactively managing program liability.
- Customer insight and personalization – becoming able to make accurate decisions based on individual customers’ profiles rather than on broad segments. This will allow brands to build greater affinity by anticipating customer objectives and being present when the customer needs your help.
- Making loyalty mechanics more efficient and effective – by controlling costs and focusing investment on the most valuable customers, so that loyalty investment is more impactful on the bottom line. This will also help brands optimize their performance in environments they don’t control – like third-party marketplaces.
- Problem solving & prevention – around operational issues, admin errors, customer errors and fraud. If you can anticipate a big problem and deal with it in a timely manner, then most issues can remain small and possibly not even be noticed by the business or customers.
Progress in all of these areas, for artificial intelligence, has been constrained by available technology until the past 6-12 months. Legacy technology constraints can still impede progress, but most companies have modernized their tech stack sufficiently in the past three years, that even legacy systems are no longer a roadblock.
In many cases AI is still constrained by the quality of the data, or the quantity of the data needed to train algorithms. As the saying goes, ‘garbage in, garbage out’. Few travel companies have a problem with the quantity of data, but given the many business units in a typical travel company, getting all the right data into a single, logical repository to let AI do its job, remains elusive.
This is actually more of an organizational problem than a technology problem – and one around which business leaders appear to be making good progress. Brands, therefore, should be aware of the potential applications of the technology now and start experimenting with low-cost, third-party solutions, so they can capitalize on opportunities around artificial intelligence as they arise.
Using AI to improve profitability of pricing and promotions
Revenue management and the loyalty team have often been at odds since loyalty programs were created.
The loyalty team wants access to inventory to use as rewards and incentivize engagement. The revenue management team wants to generate cash from available inventory. Today, revenue management systems know 30 days in advance, probably within one standard deviation, how many seats will be empty on any given flight, or how many hotel rooms will go unsold – and so both teams can work more proactively together, to optimize value for the company from that available inventory.
When revenue management works closely with the loyalty team, they can arrange for those seats or rooms to be sold at special prices or as part of dynamic packages. This can create incremental revenue, and/or reduce loyalty program liability, and is key to delivering high perceived value to the customer, at low direct cost.
What’s missing at most businesses is a way of calculating the ideal level of inventory to release as rewards, and at what redemption value, for greatest overall profitability. Businesses estimate this based on historical data on occupancy levels and other inputs, but that is challenging without AI.
AI is needed to create the algorithms and models to forecast optimal scenarios to put that inventory to use. The profitability of releasing a seat or room at a given price will vary as the date approaches, and will also be affected by factors as wide-ranging as the profile of the customer receiving the offer, and even the weather forecast for the date of travel.
With brands making thousands of items of inventory available, there is significant scope for improved profitability if AI-based models were used to allocate inventory and determine reasonable internal transfer rates. In this way, AI can alleviate one of the greatest areas of tension within the overall company, while improving efficiency and value creation.
Similar applications are possible in banking, retail, telecom, and for utilities companies to better optimize sellout among participants in the loyalty program, while increasing value for all stakeholders.
AI can be similarly beneficial in the management of distressed inventory for other types of retail companies.
Most companies have aging inventory or underutilized staff. The obvious way to avoid a total loss is to discount the distressed inventory. This practice has gone on for hundreds of years with baked bread left over at the end of the day, or fruit/vegetables reaching an advanced stage of maturity. But, it also exists with toys left over after Christmas. Enabling customers to redeem or buy at better value is a great way to create incremental value.
Brands can also package distressed inventory with other products or services, at a price attractive enough to find a buyer, without the customer or competitors knowing exactly what they paid for the room, flight, or other goods in the bundle.
Products combined in this way, in the travel sector, are known as dynamic packages; these have been around for a long time. AI can enhance this opportunity on a unit-by-unit basis, using sales channel insight and customer insight to fill this capacity with a positive contribution margin.
Yield management has matured greatly in the past decade, in part enabled by AI solutions. Greater investment in AI will continue to help tackle constraints placed on inventory by market factors, such as the challenge described above.
Regarding the management of points liability: loyalty programs with more optimized data models and engaged customers would be able to put together special offers, which might include dynamic packages or bundled offers to get the majority of points/miles redeemed for distressed inventory. This is the most powerful tool that AI can unlock for improving profitability across the business.
The key is enabling customers to redeem points with a much higher perceived value than the actual cost of delivery.
Improving customer insight and loyalty personalization
During the past 5 years, many airline loyalty programs have proclaimed they intend to become lifestyle businesses. That is a wise recognition of the fact that if they don’t make this leap, they will become commoditized.
Being a lifestyle business would imply being valued by the customer around their personal interests and goals – rather than their functional need. In travel, that would imply engaging customers around their reasons for travel, rather than just providing the seat on a plane or the hotel room. However, few are advancing rapidly in this transformation.
Airlines claim to have significantly improved personalization over the past five years, but in my opinion even if they are twice as good as they used to be, they are still only 5-10% along the journey to true personalization at the individual or small-segment level. I receive dozens of emails from airline loyalty programs each month, and to be honest, none of them feel personalized in spite of the significant data most of the airlines have about me. There is an enormous opportunity for AI and machine learning to help identify engagement opportunities and craft personalized content.
The travel industry is an excellent sector to test and use AI and machine learning because of the massive amount of data, and the uniqueness of each business or leisure trip.
People rarely travel because they like to sit in an airplane seat for 2-12 hours, or deal with airport security. Fundamentally, people travel for only two reasons:
- to communicate more effectively by being face-to-face
- to expand their personal horizons – by engaging with a distant culture, seeing sights in person that are more stimulating than viewing photographs, or simply to enter an environment that is different than their day-to-day routine.
Very rarely do two trips have the same objectives, involve the same people, or comprise the same modes of transportation. This is remarkable given that over a billion flights are booked each year. Given the infinite possible combinations, only AI has the potential to help travel companies help their customers achieve their personal goals.
In this context, instead of the traveler or their travel agent searching around 20 to 200 alternative options for a given trip, AI could do much of the analysis so the traveler can simply choose one of the 3-4 best alternatives presented.
This has huge ramifications for search engines like Google, or OTAs like Booking.com, Trip, or Expedia – because intelligent agents evaluating the travel options don’t engage with advertising. They just logically and rationally combine the best components and present them with no emotion involved. This means people search less, and simply choose their preference.
For the travel companies like airlines, helping customers achieve their travel goals can transform their business from a company that moves large aluminum tubes from one airport to another, into a problem-solving partner that builds affinity with their customers.
Beyond booking trips, AI has the potential to improve the targeting and personalization of a wide range of other brand engagements, and increase the utility of data that these interactions yield.
In this context, data that can be obtained from loyalty program partners will often signal a customer’s true lifestyle preferences and the degree of their disposable income. Adding partners that issue your points/miles in complementary spending categories is perhaps the most effective manner to capture data that can be fed into profiling algorithms.
Static redemption catalogs, with content curated and aggregated as rewards, are evolving into redemption marketplaces – where content is dynamic. With the help of AI, the pricing can also become dynamic. It is not difficult to imagine an algorithm determining what mix of product- and service-based redemptions can optimize the cost of goods sold against their perceived value to the customer, in order to drive conversion.
Gamification is another area which stands to be improved by AI. There are thousands of game technics that can be deployed, but not all customers respond to incentives in the same way – so some will work better on some segments than others. AI has the potential to figure out which types of stimuli are most appropriate to which individuals as the data model improves and the algorithms learn from trial and error.
Offering a prize is usually the most effective way to get a person to perform an action, but most customers are smart enough to calculate whether the effort is worth the possibility of earning the prize. AI can adjust the factors in this equation to make sure the results are worthwhile for the airline and loyalty program, and that the offer is calibrated appropriately to the individual targeted.
Currently, brands often deploy gamification as a means of collecting customer insight. But brands who continue to invest in AI will find richer insights waiting to be uncovered in their existing apps and other digital environments – once they gain the tools and expertise to collect, process, and understand the data.
Making loyalty mechanics more efficient and effective
Historically, targeting in loyalty programs has relied on fairly general insights into the behaviors and preferences of broad customer segments – but the potential for growth based on this kind of targeting is limited. The next phase of optimization will rely on more accurate targeting across smaller segments based on all the available and relevant data.
“Available relevant data” is an important concept. Companies have lots of data, but most of it is just noise. AI can help identify which data has high correlation to specific outcomes. Learning which data matters will help with collection. It will almost certainly demonstrate that data from partners, interacting with your customers, will complement data from your own customer touchpoints, dramatically improving the profiling that in turn improves personalization.
Customer segmentation is already improving where AI and machine learning have been applied and will only get better as the algorithms improve over time. This allows companies to better understand ROI by customer segment, and I suspect has led to the realization that a good deal of profit, and probably more upside potential, exists in the mid-tail and longer-tail segments, i.e., those less frequent customers who allocate share-of-wallet across competitors.
Loyalty marketing professionals have long focused on their premium customers – with good reason, but allocating 80% of the budget to satisfy 10-15% of total customers has not produced the best results. People in the second quartile are probably spending significantly in a particular category, but they allocate their spend across suppliers for various reasons. When a retailer can identify those people and incentivize them to allocate more share of wallet, benefits can increase for all stakeholders.
Data from partners of the loyalty program can be invaluable in determining a person’s real lifestyle interests, and so most types of businesses should expand their mix of partners to include a few in each spending category. This will allow them to capture more insightful data, based on customers earning or redeeming points among those partners.
Today in airline loyalty programs, most partners are the same big hotel groups, rental car companies, and tour and activity operators – but those services are only useful to a customer when they are traveling. Since most people travel by air less than two times per year, a loyalty program needs to capture data from partners that are relevant to these people every week or month. Those potential partners are non-travel companies.
A customer who earns points/miles from a fuel retailer where they put premium gas in their car at least once per month is far more likely to respond to upgrade offers – because they obviously have discretionary income. A person earning points/miles from a coffee shop chain 2,000km from home, but who did not get there with your airline, is probably not allocating 100% share of wallet. And there is a big difference between someone who buys 1 shirt for £200 at Harvey Nichols rather than a person who buys 4 shirts for £200.
Of course, AI algorithms will need to train on partner data, but the resulting models will indicate how to best engage with segments whose interests are more varied and complex than those of business travelers. Longer-tail customers, earning hundreds of dollars per year in points purchased by partners, can in fact be highly profitable, since the airline is earning an attractive margin when selling the points/miles to the partner.
As mentioned, when these data models get better, AI also has the potential to identify which pieces of data have the strongest correlation with customer behavior, and help to determine which data insights are most worth collecting.
Presuming a loyalty program could eventually get 30% of data insights from their direct interactions with customers and 70% of insights from partners, they would be able to optimize the economics around partner management. I believe some data from some partners would be so valuable that an airline loyalty program might want to give partners the points/miles in exchange for such information, since that information could be monetized at a higher level than simply selling the points/miles.
It’s also worth mentioning that AI can be used to improve the status matching which airlines are already using to identify potentially valuable customers in the long tail. Travelers who frequently match their status across airlines or hotel groups can turn out to be fickle individuals that don’t actually generate the anticipated ROI. AI can once again look beyond the obvious data to identify behavioral patterns that indicate who is worth pursuing and who might actually be expensive to acquire.
Beyond engaging the mid-to-long-tail customer, the ability to process detailed customer data in this way will also be useful for tackling other challenges common to loyalty programs.
One of these is churn, where customers have been in the same tier for an extended period of time, but their lifestyles, jobs, and priorities have changed. This happened at large scale when few people flew during the covid-19 pandemic. By processing a wider range of insights on customers, AI can help identify which are at risk of churning, and which are worth nurturing in order to regrow the customer relationship.
Finally, AI can help loyalty programs to increase a brands’ footholds in third-party environments. This already takes place in some loyalty ecosystems – where customers are able to earn and burn loyalty currencies outside of a brand’s own environment. There are also, however, some interesting use cases of AI in sentiment analysis, whereby a brand can read and interpret publicly available content in order to identify and address customer needs more quickly.
To date, sentiment analysis has been applied mostly to comments on social media, but the travel industry has been exposed to marketplaces for decades. These were originally travel agents working offline, but since OTAs have stepped in, far more customer engagements now take place in digital environments which can more easily be monitored by brands. You can also obtain sentiments from customers by analyzing online chat sessions, reviews, or distributing surveys. This zero or first-party data can be invaluable – albeit challenging to analyze at scale without AI.
In the future, many more organizations will get involved in delivering travel solutions, and customers will spend more of their time in environments not controlled by the airline or hotel group. Sentiment analysis can be applied across these expanding ecosystems so that the travel provider can remain visible, engage with customers, solve their problems, and grow their business in marketplaces which they only lightly influence.
Solving & preventing common problems with AI
There is a very wide range of business problems, challenges and inefficiencies which could be tackled with AI. I will summarize here the major ones that apply to loyalty and aviation, but many of these would apply similarly to businesses in other industries.
Better managing fluctuations in trade
Many capital-intensive businesses, including airlines, must staff many departments for peak demand. Peaks may only happen 5-15% of the time, which means organizational capacity is beyond the necessary level 85%+ of the time.
In aviation, these peak periods are characterized by increased workloads around routine customer problems – such as rebooking customers when they are going to miss a connection, or answering questions during moments of disruption. Currently, the complexity of these tasks means they depend on human management, but greater data processing capability of AI would allow many of them to be automated. Furthermore, AI could help to fine-tune each response by treating each customer based on their value to the business.
Minimizing human error
Even if people only make a mistake 0.1% of the time, this is still 1 mistake for every 999 correct actions. If an airline is handling 2 million touchpoints with customers every day, that is 2,000 mistakes per day – all of which affect real people trying to achieve their personal travel goals.
As discussed above, many processes and decisions, which would require too much data processing for conventional technology, could be automated with AI – or AI could be used to identify and flag human errors immediately as they occur.
Responding to business disruption
It is well documented how much money an airline can loose during periods of disruption. Some disruptions may be directly or indirectly caused by the airline itself, but the most severe and costly ones are often related to weather, volcanoes, or global health threats.
The cost of full contingency planning for such events is prohibitive, but AI can make businesses much more responsive to such operational problems and the resulting customer needs, reducing the negative impacts on the brand or the company’s financial statements.
Fraud monitoring & prevention
Third parties have been offering fraud monitoring solutions based on AI to airlines and their loyalty programs for most of the past decade. These have improved dramatically and will continue to improve – hopefully as fast as the criminals come up with new exploits.
As the IT department at airlines becomes more familiar with using AI across the business, some of the highest volume monitoring can be brought in-house to reduce external dependencies and cost.
AI can decide when a potential fraud attack is criminal in nature or a possible case of friendly fraud. It can then help the company take the most appropriate action. Historically, systems were shut down or customers/criminals were locked out when potential fraud was spotted, but most of the time, this is an overreaction. AI models can learn when to reject a transaction or simply freeze it for human review – or even when to allow it to take place because the cost of mitigation exceeds the cost of the fraud in the first place.
Importantly, when new cases of fraud are identified, the AI-based system can suggest how to respond quickly, while monitoring if the situation is an isolated event, or if it could grow into a significant threat.
Competition in AI-powered loyalty will span industries
I have been fascinated by data science and artificial intelligence for more than two decades. They are related, but different disciplines, and both showed great promise for a long time. However, both were also slow to evolve until the past five years – and especially the past 12 months. I believe this slow maturing was down to missing building blocks like low-cost, extensible cloud technologies, flexible algorithm engines, and fast enough computer processors to turn around results in a reasonable timeframe.
Today, we have cloud-based data hosting and aggregation services, such as Snowflake, that tend to be half the cost of building your own hosting environment. We also have exponentially more people who can ask the right questions about what the data may reveal – while at the same time, the algorithms have become smart enough to self-learn and can even figure out what to look for across terabytes of data.
Furthermore, I have felt that people involved with AI for most of the past two decades were more curious about what AI might be able to do, rather than focusing on use cases that maximize ROI. Today, greater business discipline, more focused business leaders, and simply more professionals that understand the technology are allowing companies to solve business problems that were too big and complicated for previous technologies.
AI has the potential to analyze data at scale, and make qualified and quantified recommendations for optimal operations. This should help departments to agree on common goals and stop fighting about priorities based on opinion, or arguing based on data from only their own silos. It should also tear down the walls that have separated business units with competing agendas, so that a more agile organization can work as one toward common objectives. Common agendas and collaboration at the organizational level can allow AI to work its magic – at scale – to deliver real ROI.
Of course, this can be a massive cultural change for any brand, but those who can evolve in this direction have the potential to convert themselves from low-margin, highly cyclical, and capital-intensive businesses, into lifestyle brands that customers can rely on to achieve their personal goals. The objective is to become indispensable to your customers.
I speculate that the majority of investment needed around AI is not in the infrastructure or algorithms, but rather in people and organizational transformation.
Such transformation sets the stage for incumbents to be replaced by a new breed of competitors – that are focused on serving customers with the best solutions to get them from point A to point B. Even in air travel, getting from point A to B is not airport to airport, but rather from the customer’s home or office to a specific destination beyond the destination airport itself.
History tells us that incumbents are often too late to adopt emerging tech. Mobile geolocation was available for some years before Uber reached widespread fame; most taxi firms simply didn’t respond fast enough.
The barriers to entry in aviation are higher, and so at present, airlines have time to expand their horizons beyond the airport, discover new opportunities to serve customers, and generate ancillary revenue. But these opportunities exist for every business that thinks about how they can serve customers beyond their traditional capabilities. Those businesses deploying AI to better understand customers’ needs will be well-placed to expand their business – and importantly, their relationships with customers.
The barriers for effective AI use are much lower today around loyalty marketing, but you need to experiment and develop a clear vision for how AI can serve your business, and help you to serve your customers.
In every industry, loyalty programs which fail to invest in AI will soon start losing members to brands they’d never previously considered a competitive threat.